Research on Embeddings for Notes
This project explores the use of embeddings to analyze and organize a collection of notes. The process involves generating embeddings for the notes, finding the closest embeddings to a given input text, and visualizing the generated embeddings.
The workflow consists of three Python scripts:
generate_embeddings.py
- Generates embeddings for notes in a specified folder.get_closest_embeddings.py
- Finds the closest embeddings to a given input text.vizualize_embeddings.py
- Visualizes the generated embeddings using PCA or t-SNE.
An example workflow can be found in the provided Python scripts section.
Findings
During this research, several observations were made:
- Storing vectors in JSON files is efficient, as it takes only about 2 seconds to find nearest vectors and write content.
- Separating notes by headings may result in less informative vectors for short headings. Adding metadata or using a two-stage approach (finding best-fitting documents first) could help improve results.
- The stored Vector Json is 25MB
- Excluding indexed notes might be necessary, as templates can interfere with queries.
Visualizations of clustered embeddings revealed some interesting patterns:
- Including filenames in the analysis seemed to have a significant impact on grouping, which may be beneficial or detrimental depending on the desired outcome.
- Removing empty files and enforcing a minimum character count (e.g., 100 characters) improved clustering quality.
- Clusters of research articles, documentation, and other content types were observed in the visualizations.
The following images showcase some visualizations from this research:
Clustering for embeddings for each seperate heading with filenames attached:
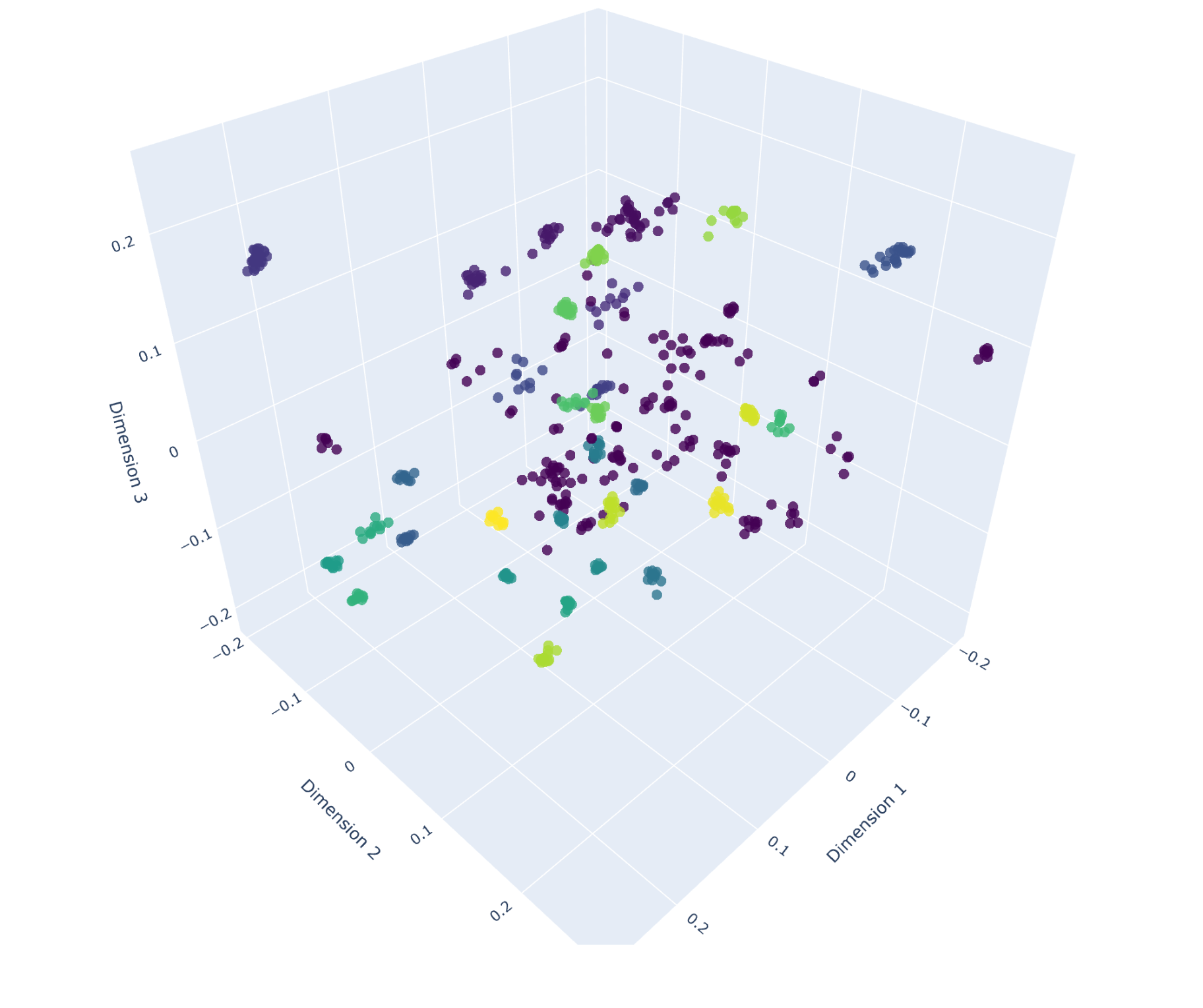
Clustered paragraph embeddings without filenames:
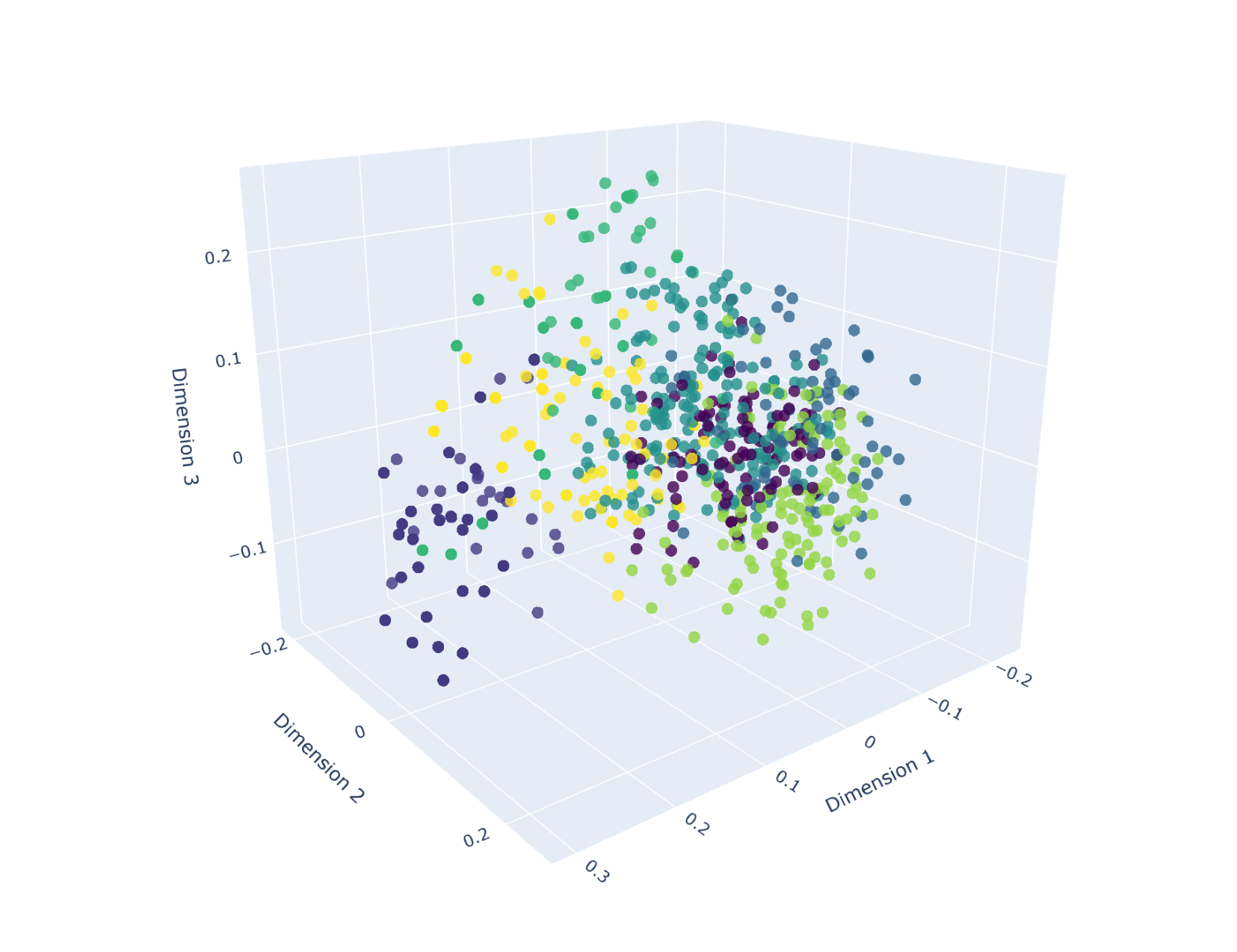
Weird Vector from empty File, Embeddings done on whole files:
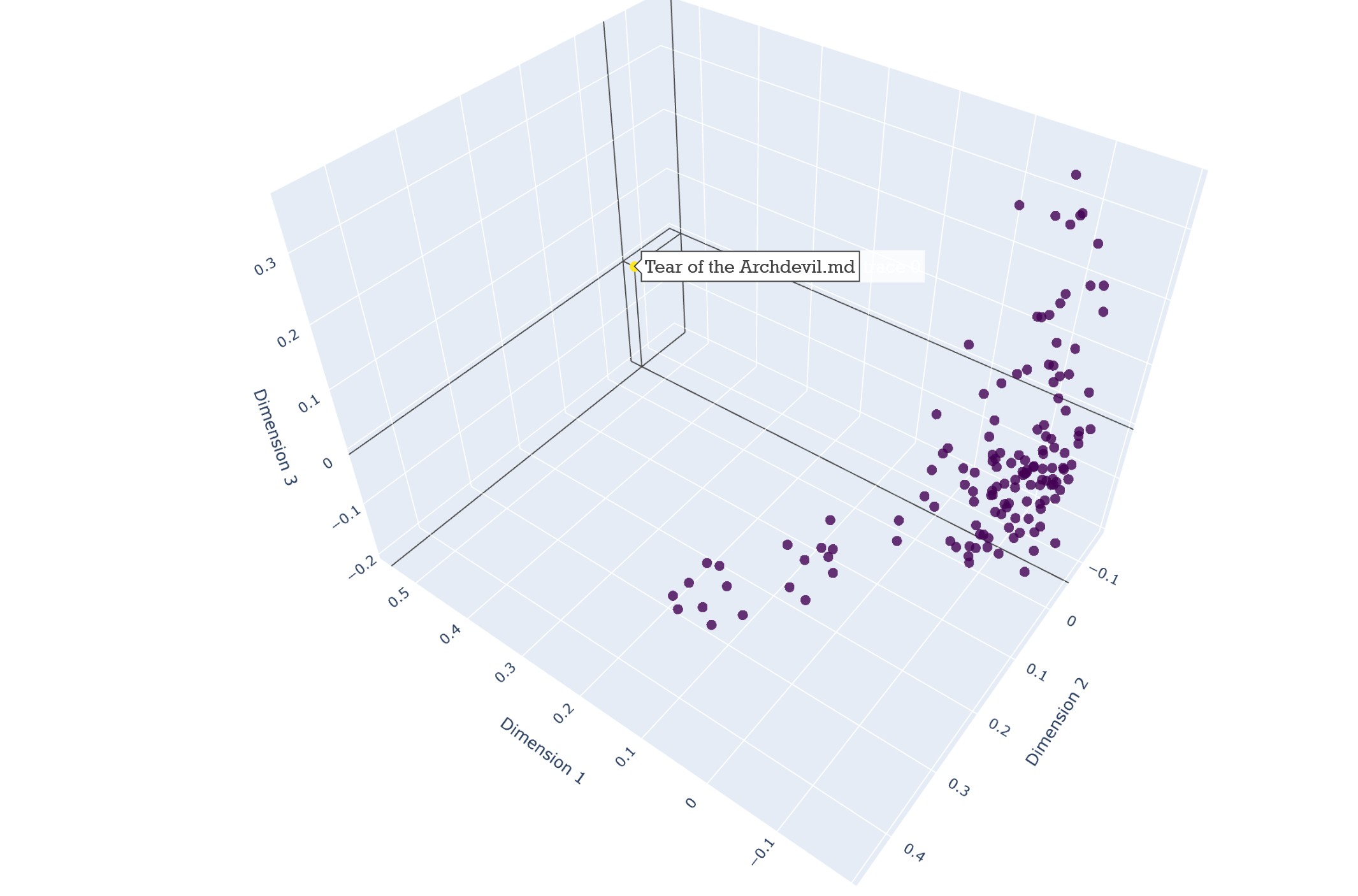
Improved clustering after removing empty files and enforcing a 100-character minimum:
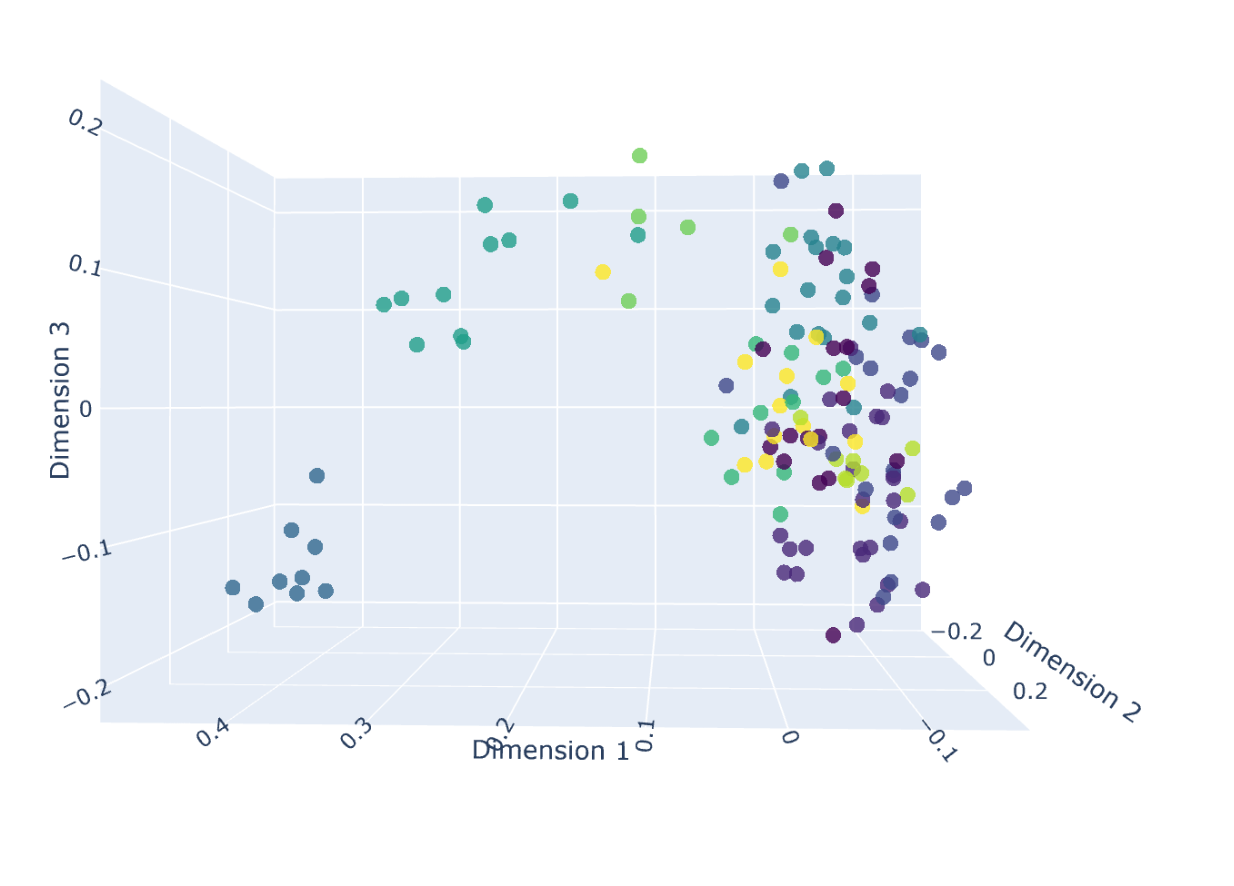
Zoomed-in view showing a cluster of research articles:
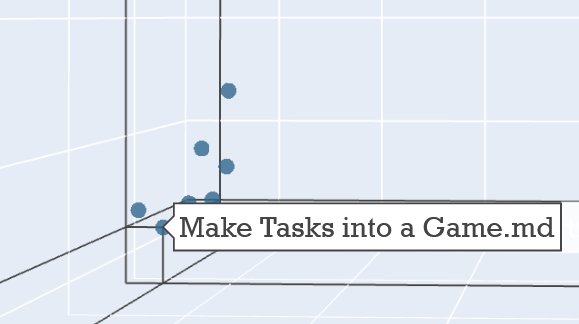
Zoomed-in view showing a cluster of documentation:
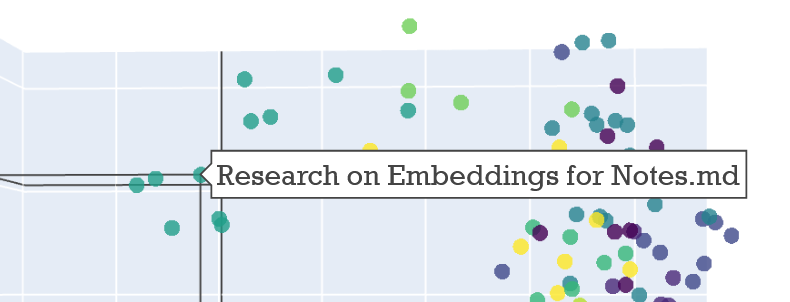
Only Fantasy Prompts:
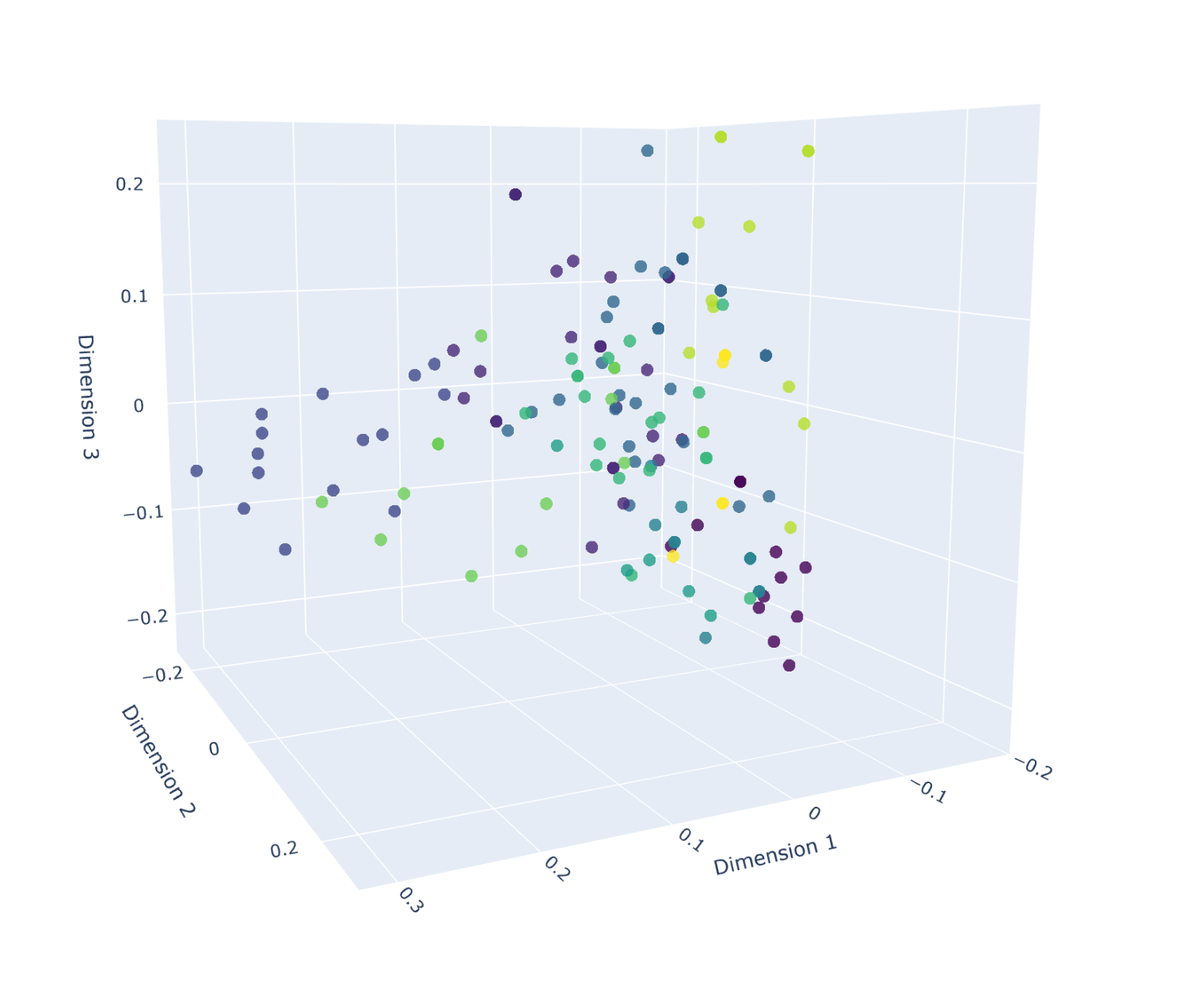
In conclusion, this research demonstrates the potential of using embeddings to analyze and organize notes, revealing patterns and relationships that can be further explored or leveraged for practical applications.
Example Usage:
As an example of practical usage, this approach was used to generate a list of interesting secrets for a fictional campaign by appending relevant prompts to an output file and running it through GPT-4. This resulted in this query output containing all important Articles: Secret Query Output File The output was appended by "Give a list of the most important Secerts of the Campain". This was the Result given by GPT-4: Important Secrets of the Campain
Python Scripts:
This project provides a set of Python scripts that help in generating, finding closest, and visualizing embeddings for a collection of notes. These scripts are particularly useful for processing and analyzing large sets of text data, such as notes or articles.
The Scripts can be found here:
1. generate_embeddings.py
This script generates embeddings for the provided notes in a specified folder.
Usage:
python generate_embeddings.py --path promised-victory --embeddings embeddings_v6.json --mode files --max_length 2000
Arguments:
--path
: The path to the folder containing the markdown files (default: 'promised-victory').--embeddings
: The path to the output embeddings file (default: 'embeddings.json').--mode
: The mode of processing - either 'files' or 'paragraphs' (default: 'files').--max_length
: The maximum length of one embedding (default: 5000).
2. get_closest_embeddings.py
This script finds the closest embeddings to a given input text.
Usage:
python get_closest_embeddings.py --embeddings embeddings.json --num_chars 10000 --input_text "this is a test"
Arguments:
--embeddings
: The path to the input embeddings file (default: 'embeddings.json').--num_chars
: The number of characters to load from the input file (default: 10000).--input_text
: The input text that should be used to find the closest embeddings (required).
3. vizualize_embeddings.py
This script visualizes the generated embeddings using dimensionality reduction techniques with PCA and clustering with KMeans.
Usage:
python vizualize_embeddings.py --embeddings embeddings.json
Arguments:
--embeddings
: The path to the input embeddings file (default: 'embeddings.json').
Example Workflow
- Prepare a folder with markdown files containing your notes, e.g., 'promised-victory'.
- Generate embeddings for these notes:
python generate_embeddings.py --path promised-victory --embeddings embeddings_v6.json --mode files --max_length 2000
- Find the closest embeddings to a given input text:
python get_closest_embeddings.py --embeddings embeddings_v6.json --num_chars 10000 --input_text "this is a test"
- Visualize the generated embeddings:
python vizualize_embeddings.py --embeddings embeddings_v6.json
Comments
We'd love to hear how you've used this content in your own campaigns! Share your anecdotes and examples in the comments below to inspire and help other readers imagine the possibilities. Your input can also help us refine and improve the note structure for future adventure modules.